Understanding Data Labeling Platforms: A Comprehensive Overview
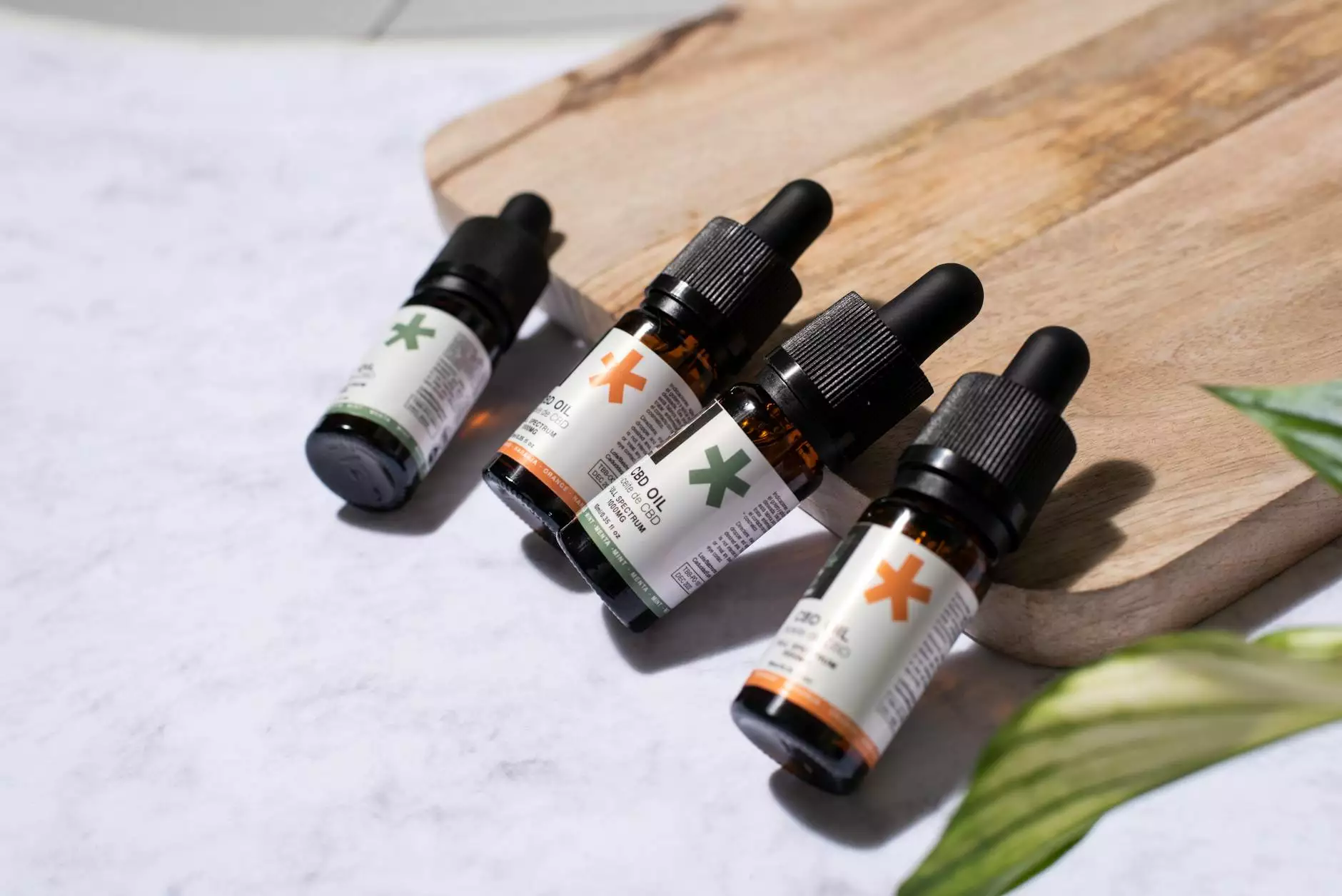
Data labeling platforms are revolutionizing the way businesses operate in various industries. As we delve into this intricate topic, we will explore the significance of these platforms, their applications, and why they are becoming crucial for businesses that aim to leverage data effectively. In a world that is increasingly driven by artificial intelligence and machine learning, the role of data labeling cannot be overstated.
What is a Data Labeling Platform?
A data labeling platform is a specialized tool that enables companies to organize, annotate, and prepare vast amounts of data for machine learning models. The essence of this platform is to provide accurate labels that can be used to train algorithms, thereby enhancing their performance in making predictions or classifications based on new, unseen data.
The Importance of Data Labeling
Data labeling is an essential step in the machine learning lifecycle. Without properly labeled data, machine learning models cannot learn effectively. Here’s why proper data labeling is crucial:
- Enhances Accuracy: Accurate labeling leads to high-performance models that can make precise predictions.
- Facilitates Learning: Well-structured datasets help algorithms learn relationships and patterns efficiently.
- Reduces Errors: High-quality labels minimize the likelihood of errors during predictions, enhancing model reliability.
- Supports Diversity: Comprehensive datasets ensure that models can handle a variety of inputs and scenarios.
How Data Labeling Platforms Work
Understanding how data labeling platforms function is key to grasping their value. Here’s an overview of the typical process:
- Data Collection: The first step involves gathering raw data from various sources, including images, text, audio, and videos.
- Pre-Processing: The data undergoes cleaning and pre-processing to remove any inconsistencies or irrelevant information.
- Annotation: This is the core of the labeling process, where human annotators or automated tools assign labels based on predefined categories.
- Quality Assurance: Rigorous checks are implemented to ensure the accuracy and consistency of the labels before the dataset is finalized.
- Model Training: The labeled data is then used to train machine learning models, enabling them to learn and make predictions.
Applications of Data Labeling Platforms
Data labeling platforms have a broad spectrum of applications across different sectors, enhancing their operational capabilities:
1. Healthcare
In the healthcare industry, data labeling platforms assist in annotating medical images and patient records. Through accurate labeling, systems can predict diseases and automate diagnosis, leading to better patient outcomes.
2. Autonomous Vehicles
Self-driving cars rely heavily on labeled data for real-time decision-making. Data labeling platforms are crucial for annotating images and video feeds, enabling these vehicles to identify pedestrians, road signs, and other critical elements.
3. E-commerce
Retail companies use data labeling to enhance their recommendation systems. By labeling products and customer reviews, e-commerce businesses can offer personalized shopping experiences that drive sales.
4. Marketing
Marketing analytics benefits from data labeling by categorizing consumer behavior data. This allows companies to target advertisements more effectively and improve conversion rates.
Choosing the Right Data Labeling Platform
With numerous data labeling platforms available, choosing the right one can be daunting. Here are some critical factors to consider:
1. Quality of Labels
The primary goal of using a data labeling platform is to obtain high-quality labels. Ensure the platform has robust quality control mechanisms to maintain accuracy.
2. Scalability
As your project grows, your data needs will expand as well. Select a platform that can handle large volumes of data without compromising performance.
3. Ease of Use
A user-friendly interface makes the labeling process smoother and more efficient. Look for platforms that streamline workflows and provide intuitive tools for annotators.
4. Customization Options
Your project may have specific labeling requirements. Choose a platform that allows for customization, whether it’s adding new categories or adapting existing ones.
5. Integration Capabilities
Lastly, the ability to integrate with existing workflows and tools is crucial. Ensure the platform can connect with your data storage and machine learning systems seamlessly.
The Future of Data Labeling Platforms
The future of data labeling is bright and full of possibilities. With advancements in artificial intelligence, we can anticipate several trends:
1. Automation
While human annotators will always be necessary, the integration of AI in data labeling is on the rise. Automated tools can speed up the labeling process, thereby reducing costs and improving efficiency.
2. Enhanced Collaboration
Future platforms will likely focus on enhancing collaboration among teams, providing features that facilitate real-time communication and feedback during the labeling process.
3. Increased Demand
As industries continue to embrace data-driven decision-making, the demand for high-quality data labeling will increase, leading to the growth of dedicated platforms.
Conclusion
In today’s data-centric world, a data labeling platform is not just a tool but a necessity for businesses aiming to harness the true power of their data. By ensuring accurate data annotation, these platforms play a pivotal role in training machine learning models that can drive innovation and efficiency across numerous sectors.
As businesses continue to face challenges related to data management, understanding the importance and functionality of data labeling platforms will be paramount. Investing in the right platform today will set the groundwork for greater successes in the future.