Harnessing Machine Learning Malware Techniques for Enhanced Cybersecurity
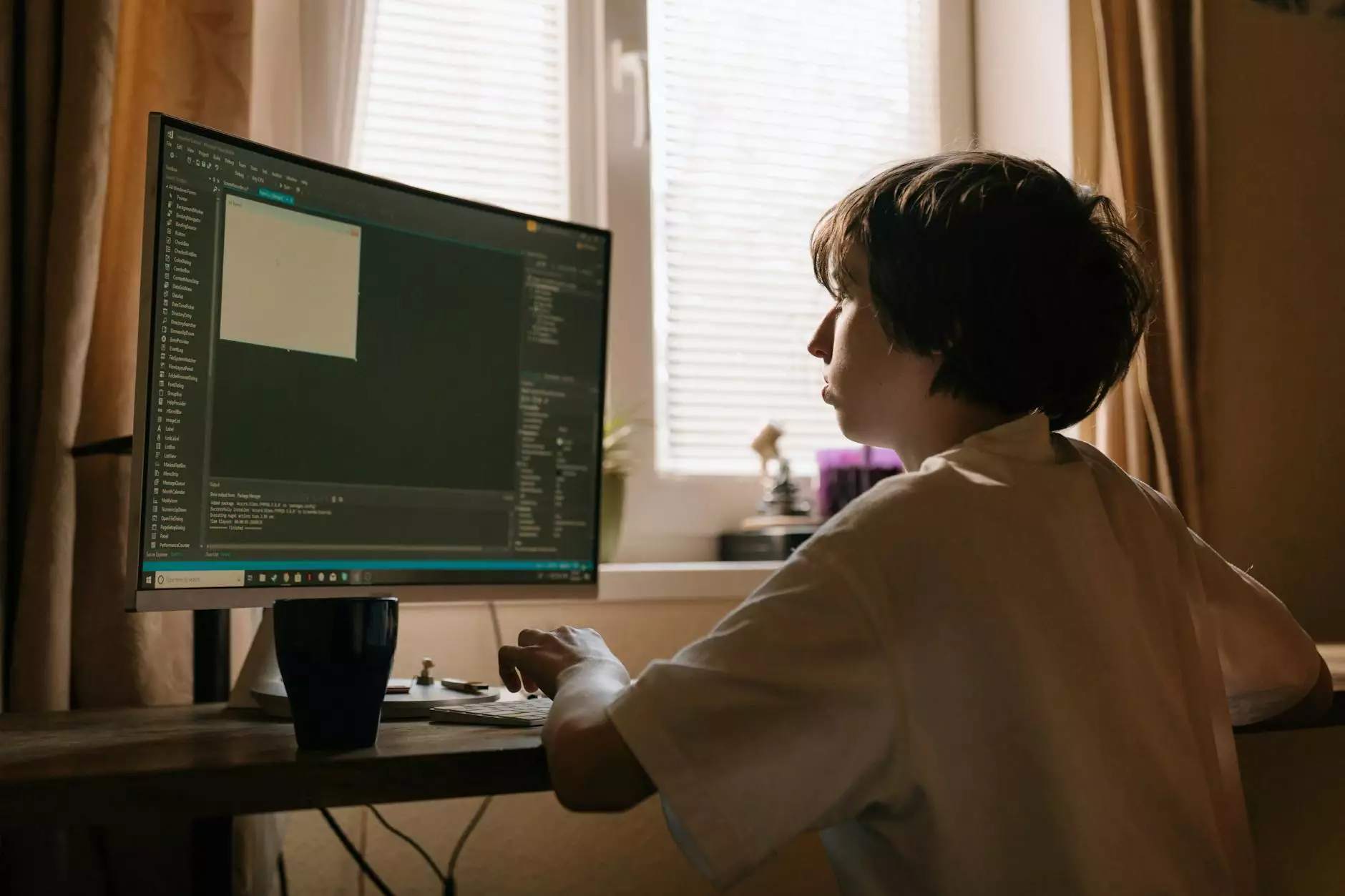
Merging technology with security has become imperative in today's fast-paced digital era. With cyber threats becoming increasingly sophisticated, traditional cybersecurity measures are no longer sufficient. One of the most revolutionary developments in combating online threats is the integration of machine learning malware detection systems. This article will explore the implications of machine learning in cybersecurity, how it helps in detecting and combating malware, and the future of security systems for businesses.
Understanding Machine Learning and Its Relevance to Cybersecurity
Before diving into the specifics of machine learning malware, it's crucial to grasp the fundamentals of machine learning (ML). Machine learning is a subset of artificial intelligence (AI) that enables systems to learn and improve from experience without being explicitly programmed. In the context of cybersecurity, ML algorithms analyze vast amounts of data to identify patterns that indicate malicious activities.
The Role of Machine Learning in Detecting Malware
Malware, or malicious software, poses a significant threat to businesses and individuals alike. Traditional antivirus solutions often rely on signature-based detection, which can be ineffective against new and unknown threats. This is where machine learning comes into play:
- Pattern Recognition: Machine learning algorithms are adept at recognizing patterns within data. By learning from historical data, these algorithms can identify the characteristics of malware, even zero-day vulnerabilities.
- Real-Time Analysis: Machine learning systems can analyze incoming data in real-time, allowing for immediate detection and response to potential threats.
- Dynamic Learning: As new malware variants emerge, machine learning systems continuously update themselves by learning from new data, ensuring greater accuracy over time.
Benefits of Using Machine Learning for Malware Detection
Implementing machine learning malware detection systems offers several benefits to organizations aiming for enhanced cybersecurity:
1. Increased Detection Rates
Machine learning algorithms tend to have significantly higher detection rates when compared to traditional systems. By analyzing various data points, they can recognize potential threats that would not be caught by conventional methods.
2. Reduced False Positives
One of the significant challenges in cybersecurity is dealing with false positives—alerts that incorrectly identify benign activity as a threat. Machine learning systems can significantly reduce these occurrences, allowing security teams to focus on genuine threats.
3. Enhanced Incident Response
With real-time threat detection powered by machine learning, incident response teams can react swiftly to contain and neutralize threats. This speeds up recovery times and minimizes the potential damage to business operations.
4. Cost-Effectiveness
Although the initial investment in advanced machine learning systems can be significant, the long-term savings are substantial. By preventing data breaches and minimizing downtime, businesses can save money and resources while protecting their reputation.
Challenges to Implementing Machine Learning in Cybersecurity
While the advantages are substantial, the integration of machine learning malware detection into existing systems comes with its set of challenges:
1. Data Quality and Quantity
Machine learning systems require vast amounts of high-quality data to function effectively. Organizations may struggle to gather sufficient data for training their algorithms, particularly if they have not previously implemented advanced cybersecurity measures.
2. Skill Gap
The deployment of machine learning solutions necessitates skilled personnel trained in both data science and cybersecurity. Businesses may face challenges in hiring or training staff with the requisite expertise.
3. Evolving Threat Landscape
The malware landscape evolves rapidly, with cybercriminals continuously developing new strategies to bypass detection. Keeping machine learning systems updated and effective against emerging threats can be challenging.
Future Trends in Machine Learning Malware Detection
The future of machine learning malware detection is promising, with several trends likely to influence the evolution of cybersecurity:
1. Integration with AI and Big Data
The convergence of machine learning, artificial intelligence, and big data analytics will provide even more robust solutions for malware detection and prevention. These technologies can work together to enhance threat intelligence and improve overall security posture.
2. Automated Threat Hunting
As machine learning algorithms become more advanced, autonomous systems capable of conducting threat hunts without human intervention will become more prevalent. This will allow for a proactive stance against cyber threats.
3. Enhanced User Privacy Protection
With growing concerns about privacy, future developments in machine learning malware detection will likely focus on ensuring that user data is protected while still allowing for effective threat detection.
4. Democratization of Advanced Security Solutions
The accessibility of machine learning technology will likely increase, allowing smaller businesses to adopt advanced cybersecurity measures usually reserved for larger enterprises.
How to Leverage Machine Learning Malware in Your Business
For businesses aiming to adopt machine learning for enhanced cybersecurity, the following steps can assist in effectively leveraging machine learning malware detection systems:
1. Assess Your Current Security Framework
Understand your existing cybersecurity framework to identify strengths, weaknesses, and areas that could benefit from machine learning integration.
2. Invest in Training and Development
Ensure your team is adequately trained to handle machine learning tools. Consider investing in professional development for existing staff or hiring new personnel with the requisite skills.
3. Collaborate with AI and Cybersecurity Experts
Partner with firms specializing in AI and cybersecurity to implement tailored machine learning solutions that fit your unique security needs.
4. Regularly Update Your System
Keep your machine learning algorithms up-to-date with the latest threat intelligence and data. Regular updates enhance the effectiveness of your cybersecurity defenses.
The Bottom Line: Embrace the Future of Cybersecurity
In conclusion, the intersection of machine learning malware detection and cybersecurity signifies a new era in the protection of digital assets and sensitive information. Businesses that adopt these advanced technologies not only enhance their security posture but also position themselves ahead in an increasingly competitive and risky marketplace. By investing in machine learning systems, organizations not only safeguard their infrastructure but also build trust with customers and stakeholders through enhanced security practices.
As we navigate through an evolving digital landscape, staying ahead of cyber threats is crucial. The marriage between machine learning and cybersecurity is not just a trend; it's a vital strategy for ensuring the safety and security of business operations.